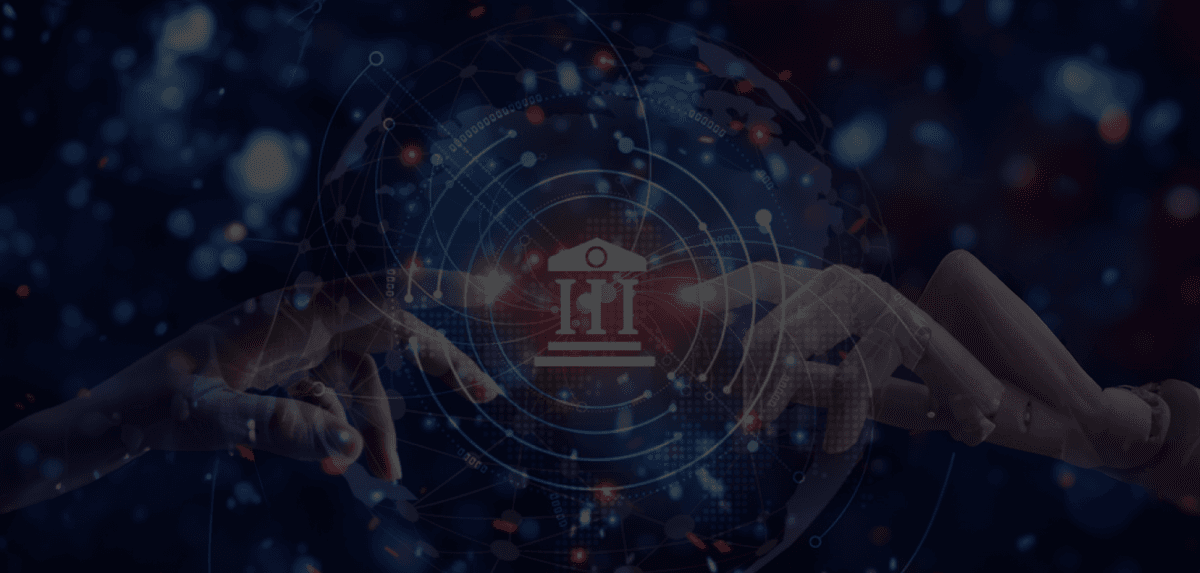
AI in Banking
Building the Bank of Future with AI
Incumbent banks face a dual challenge: achieving fintech-like speed and agility while managing the scale, security, and regulations of traditional finance. Despite investing billions in technology, few banks have successfully scaled AI throughout their operations. Here we have brought you some key challenges and AI potential in banking to operate and serve better.
Challenges in Scaling AI Capabilities in Banks
The main obstacles include a lack of clear AI strategy, weak core technology systems, and outdated operating models. High error rates; poor refresh rates, longer time to market, limited reusability of code and software across internal teams, data trapped in silos across multiple units and hard to integrate with external sources. Legacy systems often lack the flexibility and capacity needed for AI applications, and fragmented data across multiple silos hampers comprehensive analysis. Establishing a centralized data backbone is crucial for intelligent decision-making. The best-in-class data management can ensure a high degree of accuracy and a single source of truth in a cost-effective manner. it enables timely and role-appropriate access for various use cases (e.g., regulatory, business intelligence at scale, advanced analytics and machine learning. Additionally, banks need robust tools and standardized processes to build, test, deploy, and monitor AI models. Overcoming these challenges is vital for banks to effectively harness AI's potential and drive digital transformation.
AI shaping the future of banking
To successfully embrace an AI-first approach, banks need to address the challenges that hinder the organization-wide deployment of AI technologies. They should adopt a holistic approach by investing in transforming capabilities across all four layers of the integrated capability stack.
-
Engagement Layer: This layer focuses on enhancing customer interactions and experiences. Banks can leverage AI technologies to provide personalized services, improve customer engagement through chatbots or virtual assistants, and deliver tailored recommendations based on customer preferences.
-
AI-Powered Decisioning Layer: This layer involves leveraging AI algorithms and advanced analytics to drive intelligent decision-making. Banks can utilize AI techniques for risk assessment, fraud detection, credit scoring, and investment recommendations. This layer enables banks to make data-driven decisions and automate processes for improved efficiency and accuracy.
-
Core Technology and Data Layer: This layer emphasizes the infrastructure and data management capabilities required to support AI initiatives. Banks need robust technology infrastructure, including scalable computing resources and cloud platforms, to handle the processing requirements of AI algorithms. They should also prioritize data quality, integration, and governance to ensure reliable and accurate data for AI applications.
-
Operating Model: This layer focuses on organizational structure, talent, and culture. Banks need to establish an operating model that promotes collaboration, data-driven decision-making, and innovation. They must invest in developing AI talent and fostering a culture that embraces technological advancements and encourages experimentation.
By addressing these four layers of the integrated capability stack, banks can lay the foundation for an AI-first future, enabling them to leverage the power of AI technologies across the organization and drive innovation in the financial industry.